Laxmi Parida, IBM
KEYNOTE TALK – October 13
Big Data Omics: AI to TDA
I will examine the influence of computing disciplines on biology. In particular, I will talk about the use of AI and Topological Data Analysis (TDA) in the context of omics. I will also expand on a few different application of TDA in a range of problems including Covid-19.
Laura Dietz, University of New Hampshire

Laura Dietz is an Assistant Professor at the University of New Hampshire, where she leads the lab for text retrieval, extraction, machine learning, and analytics (TREMA). She organizes a tutorial/workshop series on Utilizing Knowledge Graphs in Text-centric Retrieval (KG4IR) and coordinates the TREC Complex Answer Retrieval Track. She received an NSF CAREER Award for utilizing fine-grained knowledge annotations in text understanding and retrieval. Previously, she was a research scientist at the Data and Web Science Group at Mannheim University and the Center for Intelligent Information Retrieval (CIIR) at UMass Amherst. She obtained her doctoral degree with a thesis on topic models for networked data from Max Planck Institute for Informatics. More Info: https://www.cs.unh.edu/~dietz/
KEYNOTE TALK – October 15
How to Automatically Create Relevant Articles
A long-term vision is to develop algorithms that automatically, and in a query-driven manner, retrieve materials from the Web and compose comprehensive articles that are akin to Wikipedia articles. Especially for information needs, where the user has very little prior knowledge, the web search paradigm of ten blue hyperlinks is not sufficient. Instead, the goal is to recycle Web materials with the help of Knowledge graphs to produce a comprehensive overview. While natural language generation methods like GPT-3 dominate the news, a deeper question is how to identify relevant content, what concepts are important to discuss, and how identify and organize relevant subtopics. I will present the latest findings on the way towards developing retrieve-extract-and-generate information systems.
Michael A. Bender, Stony Brook University
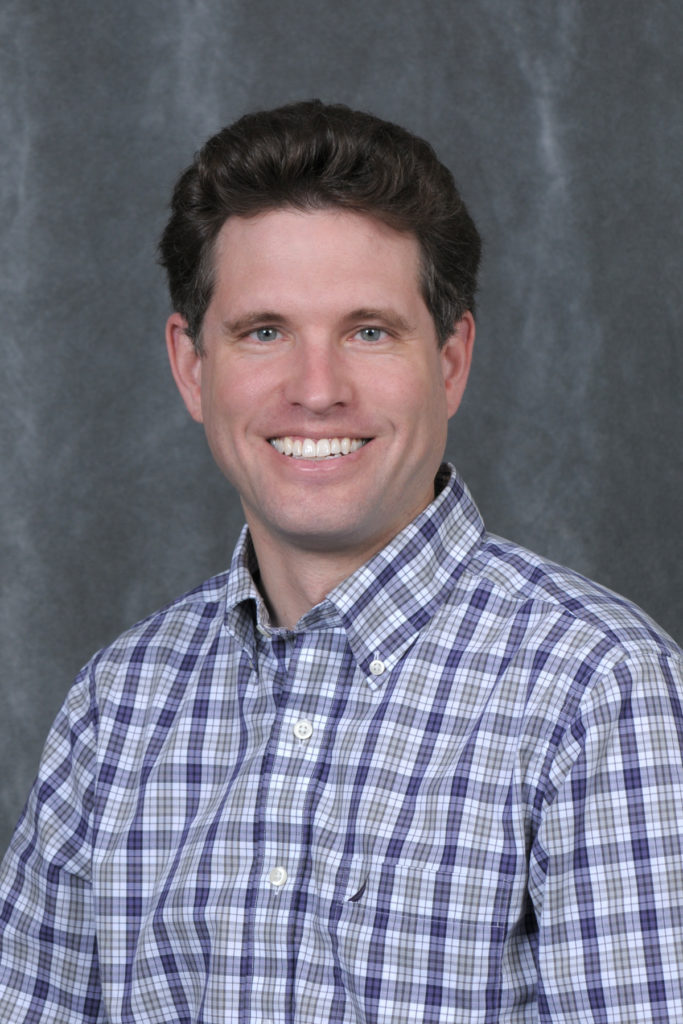
Michael A. Bender is the David R. Smith Leading Scholar of Computer Science at Stony Brook University. His research interests span the areas of data structures and algorithms, I/O-efficient computing, parallel computing, and scheduling. He has coauthored over 150 articles on these and other topics. He has won several awards, including an R&D 100 Award, a Test-of-Time award, two Best Paper Awards, and five awards for graduate and undergraduate teaching.
Bender was Founder and Chief Scientist at Tokutek, Inc, an enterprise database company, which was acquired by Percona in 2015. He has held Visiting Scientist positions at both MIT and King’s College London.
Bender received his B.A. in Applied Mathematics from Harvard University in 1992 and obtained a D.E.A. in Computer Science from the Ecole Normale Superieure de Lyon, France in 1993. He completed a Ph.D. on Scheduling Algorithms from Harvard University in 1998.
KEYNOTE TALK – October 14
Filters
A Bloom filter maintains a compact, probabilistic representation of a set S of keys from a universe U. The price of being small is that there is a (bounded) probability of false positives. This talk reviews alternative filter designs, focusing on quotient and cuckoo filters. These newer filters are faster, more space efficient, and support a broader range of operations. We focus on both the theoretical and engineering issues that arise.