Feed-Fight-Mate (FFM) is built
on top of the YAES simulation framework. The figure below
shows a screen-shot of the Feed-Fight-Mate game. Each player's
location and sensor range are represented by a cross-hair
surrounded by a rectangular box respectively. The small dots
in the environment represent energy resources and the black,
irregularly shaped, objects represents obstacles that players
cannot traverse.
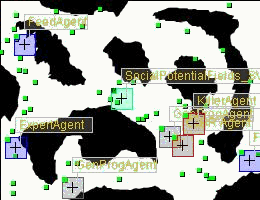 |
Screen-shot of the Feed-Fight-Mate
game with five different agent implementations. Agents
are represented by cross-hairs surrounded by a rectangular
area depicting sensor range. The small dots on the map
represent energy resources and the irregular black areas
are obstacles. |
In essence, Feed-Fight-Mate is a turn-based strategy game
with multiple paths to success. The game's objective may depend
on simulation cycles, available energy resources, agent activity
or any combination of the aforementioned components. Scenarios
that are dependent on simulation cycles can be used to evaluate
an agent's ability to explore environments, gather as much
energy resources as possible or to find landmarks within limited
number of simulation cycles. Resource based scenarios may
consist of an agent's ability to survive indefinitely in the
environment. Perhaps the most interesting agent activity scenario
to simulate is when agents are allowed to attack other agents.
|
|
Here, a wide range of attack strategies
may be employed and compared against each other. For instance
a deceptive agent may lure, simply by acting scared or by
attacking from carefully selected directions, a victim agent
into corners and then attack the victim agent hoping that
the victim agent will panic or flee. With limited freedom
of movement the deceptive agent now have a clear advantage.
Another very interesting scenario appears when agents are
allowed to mate. If too many offspring is produced then there
will not be sufficient energy resources available to keep
the population healthy. Therefore, agents will die and the
population will decrease to the extent where energy resources
are available again.
The game can be configured to exhibit many different scenarios.
Although the agent specific features and environmental objects
are configurable they still fulfill the same general purposes
in all scenarios. The game and its environment consist of
obstacles, energy resources and agents. Obstacles can not
be traversed by the agents. Energy resources are edible objects
for the agents, the energy resource serves as fuel or simply
a scoring mechanism depending on the scenario setup. The agents
are equipped, by default, with a basic set of actions, some
level of deliberative and implicit intelligence and sensors.
The set of actions consists of eating, mating, attacking,
moving and fleeing. The deliberative intelligence is in essence
a path-planner which searches for the optimum moving directions
to a local reference point if such path is existent. The implicit
intelligence consists of helper methods from which an agent
can retrieve feedback of the success of the previously performed
action and if the agent is located in an attacking position
or an eating position. The sensors are used to locate food,
obstacles and other agents. The game is turn-based; each agent
performs exactly one action per simulation cycle. The main
loop performs agent actions in a round-robin fashion.
|